In today’s evolving landscape of conversational AI, chatbots have become essential tools for customer service, information dissemination, and user interaction across industries. However, traditional chatbots often fall short of providing accuracy and contextual relevance.
The implementation of Retrieval Augmented Generation (RAG) offers a transformative solution, enhancing chatbot performance to unprecedented levels.
This article delves into how RAG improves chatbot accuracy and relevance, outlining the steps for effective implementation and the key benefits it brings.
Limitations of Traditional Chatbots
To fully appreciate the value RAG brings to conversational AI, it’s essential to first examine the limitations of conventional chatbot systems:
- Restricted Knowledge Bases: Traditional chatbots depend on pre-programmed responses or static databases, which limits their capacity to offer current or comprehensive information.
- Contextual Misinterpretation: Many chatbots struggle with understanding nuanced user queries, resulting in inaccurate or irrelevant answers.
- Inadequate Response to Complex Queries: Rule-based systems often falter when faced with multi-layered or ambiguous questions, failing to provide meaningful responses.
- Lack of Flexibility: Traditional chatbots require manual updates to adapt to new information or evolving user needs, hindering their ability to remain current.
RAG as a Solution to Chatbot Limitations
Retrieval Augmented Generation bridges the gap between static responses and dynamic, context-aware conversation by leveraging the power of large language models (LLMs) and efficient information retrieval. Here’s how RAG addresses the inherent shortcomings of traditional chatbot systems:
- Dynamic Knowledge Access: RAG enables chatbots to tap into extensive knowledge bases, providing up-to-date and highly relevant information.
- Enhanced Contextual Understanding: By integrating retrieval systems, RAG-powered chatbots can better understand the context behind user queries, offering more precise and targeted responses.
- Complex Query Handling: The fusion of retrieval and generation allows RAG systems to navigate complex, multi-faceted questions with greater accuracy and depth.
- Adaptability and Flexibility: RAG systems can easily incorporate new information into their knowledge bases, allowing chatbots to remain agile and responsive in ever-changing environments.
Implementing RAG in Chatbots: A Strategic Approach
Construct a Robust Knowledge Base
The foundation of an effective RAG system lies in its knowledge base. To ensure its strength:
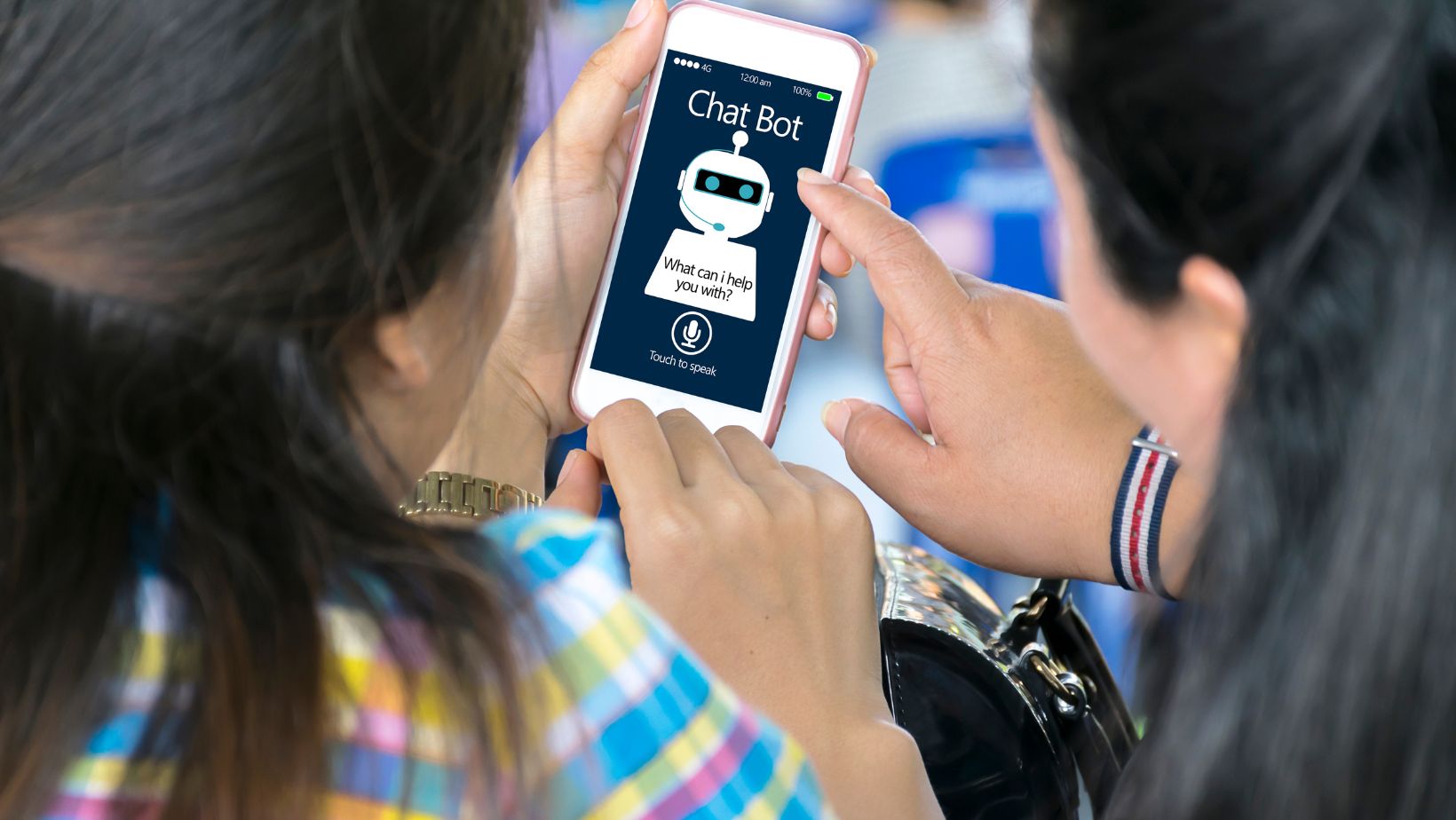
- Aggregate relevant documents, FAQs, product information, and other key data sources.
- Cleanse and preprocess this data to maintain high quality and consistency.
- Utilize a suitable embedding model to convert textual data into vector embeddings for easier retrieval.
Select a High-Performance Retrieval System
Choosing the right retrieval system is critical for real-time efficiency:
- Implement a vector database optimized for large-scale similarity searches.
- Employ indexing techniques, such as Hierarchical Navigable Small World (HNSW), to ensure rapid retrieval.
- Ensure the system supports real-time updates to accommodate dynamic data.
Fine-Tune an Appropriate Language Model
Selecting and optimizing an LLM for your chatbot is equally important:
- Factor in model size, performance capabilities, and specific deployment needs.
- Fine-tune the LLM on domain-specific data to ensure that responses remain highly relevant and contextually appropriate.
Integrate Retrieval and Generation Systems
To bring retrieval and generation together in a cohesive RAG framework:
- Convert user queries into vector representations to facilitate efficient information retrieval.
- Retrieve relevant data from the knowledge base by assessing vector similarities.
- Combine the retrieved information with the original query to enrich the context.
- Utilize the LLM to generate a response based on this augmented context.
Implement Post-Processing for Response Optimization
Ensuring the quality of chatbot responses involves post-processing steps:
- Introduce filtering and ranking mechanisms to ensure responses are coherent, relevant, and aligned with user expectations.
- Utilize confidence scoring to determine when to fall back on pre-defined responses or escalate to human operators.
Design an Intuitive User Interface
A user-friendly interface is key to fostering seamless interaction:
- Ensure a smooth conversational flow, allowing the chatbot to handle natural language input effectively.
- Provide interactive features like clarification prompts or topic-switching options to manage complex or evolving conversations.
Establish Monitoring and Continuous Improvement Systems
Ongoing refinement is essential for maintaining high chatbot performance:
- Set up robust logging and analytics tools to monitor chatbot performance and track user satisfaction.
- Develop feedback mechanisms for users to report any issues, inaccuracies, or unsatisfactory responses.
- Regularly update the knowledge base and fine-tune the system based on user feedback and emerging information.
The Benefits of RAG-Enabled Chatbots
Implementing RAG in chatbots yields numerous advantages, significantly improving both user experience and system performance:
- Greater Accuracy: RAG enables chatbots to deliver more accurate, up-to-date responses by accessing dynamic external data.
- Increased Relevance: By understanding the context more effectively, RAG-powered chatbots offer responses that are more relevant and aligned with user needs.
- Complex Query Handling: RAG allows chatbots to address multi-dimensional queries, providing more comprehensive and thoughtful responses.
- Minimized Manual Updates: The dynamic nature of RAG systems reduces the need for frequent manual updates to the chatbot’s knowledge base.
- Enhanced User Satisfaction: Accurate and relevant responses foster a more satisfying user experience, increasing trust and engagement with the chatbot.
- Scalability: RAG systems are built to handle growing knowledge bases without sacrificing performance, making them highly scalable for expanding business needs.
Challenges and Considerations in RAG Implementation
While the benefits of RAG are significant, there are challenges to be mindful of:
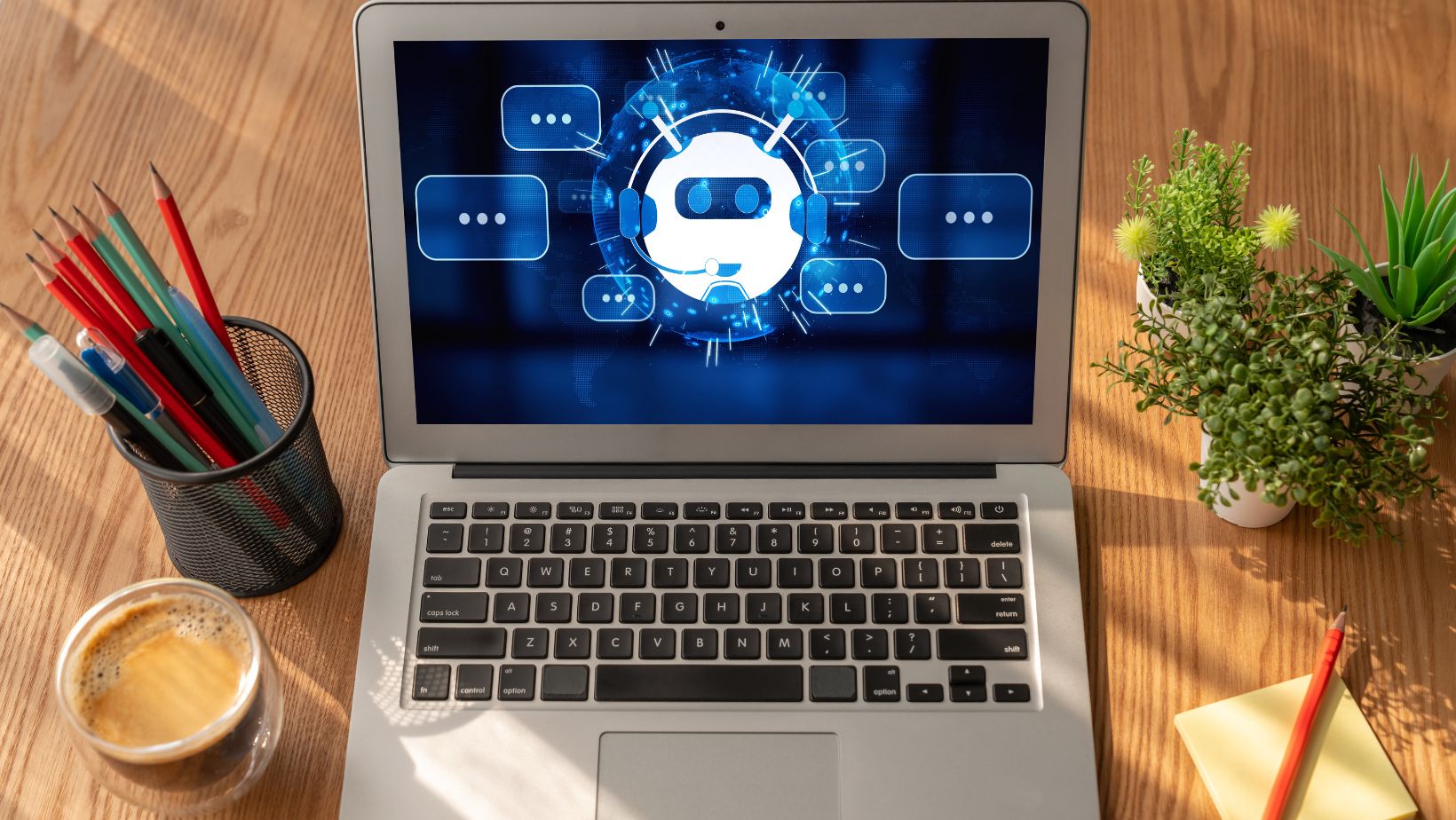
- Resource Demands: RAG systems may require more computational resources than conventional chatbots, particularly for real-time applications.
- Data Privacy Concerns: Compliance with data privacy regulations, such as GDPR or CCPA, is crucial when handling sensitive information.
- Balancing Retrieval and Generation: Finding the optimal balance between retrieved data and generated content is key to ensuring coherent responses.
- Ambiguity Management: Systems must be designed to manage ambiguous or contradictory information within the knowledge base effectively.
A New Era in Conversational AI
The integration of Retrieval Augmented Generation into chatbot systems represents a major advancement in conversational AI technology. By combining the expansive knowledge access of retrieval mechanisms with the contextual understanding of large language models, RAG-powered chatbots offer unprecedented accuracy, relevance, and adaptability.
As organizations increasingly look to improve user engagement and streamline information delivery, RAG presents a compelling solution. While challenges remain, the potential for enhanced accuracy, relevance, and user satisfaction makes RAG a strategic investment for any enterprise seeking to elevate its chatbot capabilities.
With ongoing advancements in RAG technology, we can expect the future of conversational AI to become even more sophisticated, transforming the way businesses and users interact in the digital age.